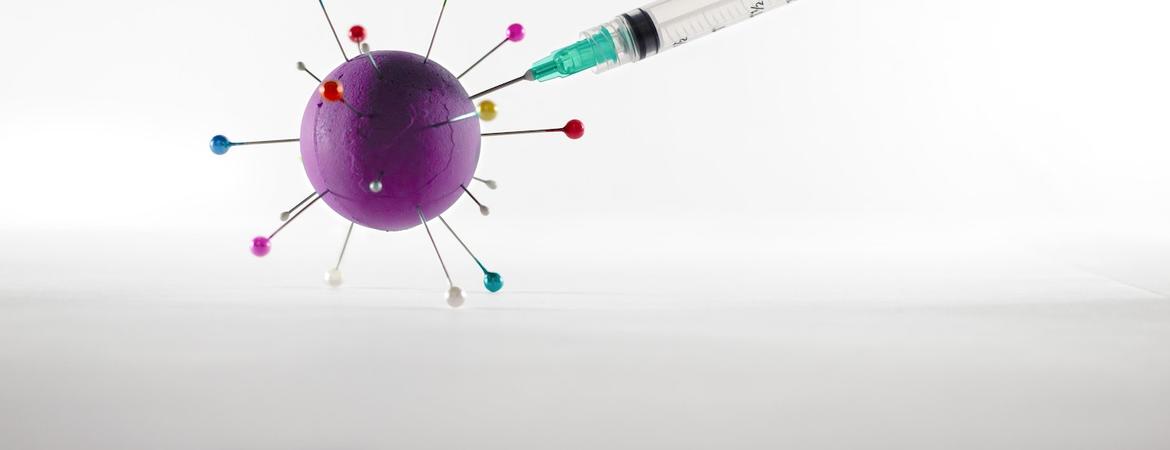
New pathogens like COVID-19 might soon face an army of custom-made antibodies designed by artificial intelligence technology that was originally developed to produce deepfake images and videos.
A consortium of scientists from UC Riverside, the Keck Graduate Institute, and Pomona College will use a family of deep learning neural architectures called generative adversarial networks to predict the structure of antibodies that could neutralize a previously unknown antigen or a new virus that might emerge in the future.
The project has received $3.2 million in funding over five years from the National Institutes of Health’s Directorʼs Transformative Research program. This fund supports inherently risky and untested projects that can potentially create or overturn fundamental science, technology, and medicine paradigms.
“While the focus of the project is on COVID-19, our framework could be used to study the immune response to other infectious agents,” said Stefano Lonardi, a professor of computer science in UC Riverside’s Marlan and Rosemary Bourns College of Engineering.
Jeniffer Hernandez and Animesh Ray at KGI, and Matthew Sazinsky at Pomona College, will use a new molecular biology technique that takes advantage of single-cell DNA sequencing to measure the antibody response against injected antigens in mice, in this case fragments of the spike protein of SARS-CoV-2, the virus that causes COVID-19.
Lonardi’s lab, in collaboration with Ray’s lab at KGI, will devise new machine-learning methods that can learn from the mouse data which antibody chain sequences can bind tightly to the antigens. To do this, they will use generative adversarial networks they believe can discover associations between the binding site on an antigen and the variable chain features of the antibody.
Generative adversarial networks are two neural networks that challenge each other in a zero-sum game, where one agent's gain is another agent's loss. This competitive game allows the neural networks to learn from the data, but also to generate new data with the same complex patterns and statistics as the training set.
Generative adversarial networks are mostly used in image and video processing. For example, special effects artists and researchers use one network to detect deepfake videos generated by another network, which “learns” from its mistakes and produces more realistic subsequent videos.
The group believes that generative adversarial networks will help them discover the structures of antibodies that would be able to halt future pandemics with faster vaccine development and rapid, tailor-made monoclonal antibody treatments.
“To the best of my knowledge, generative adversarial networks have never been employed in computational immunology,” Lonardi said.
Beyond infectious disease, the method could predict antibodies that neutralize proteins made by cancer cells, or help people manage allergies and hypersensitivity issues.
About $700,000 will be awarded to UCR to train graduate students who will execute the project.