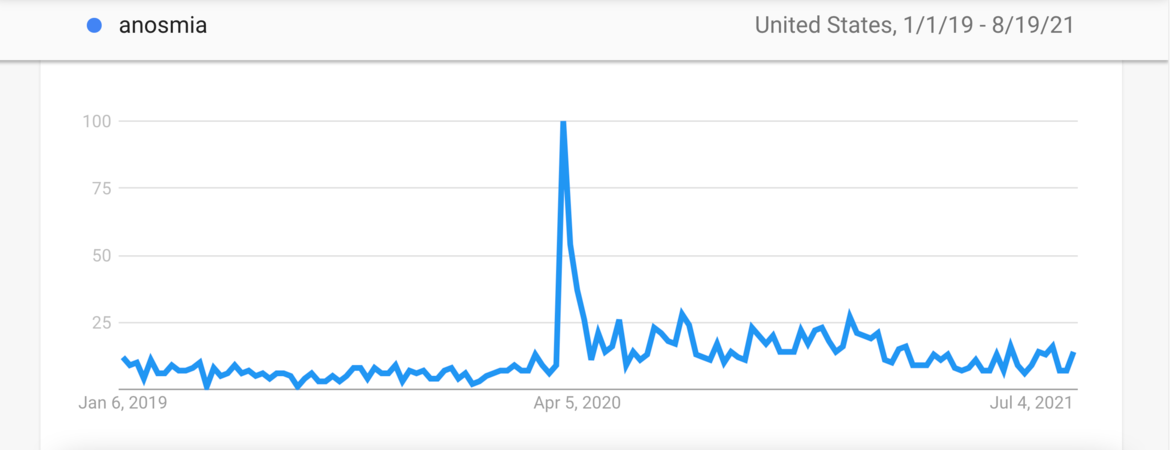
UC Riverside computer scientists are developing tools to help track and monitor COVID-19 symptoms and to sift through misinformation about the disease on social media.
Using Google Trends data, a group led by Vagelis Papalexakis, an associate professor in the Marlan and Rosemary Bourns College of Engineering; and Jia Chen, an assistant professor of teaching, developed an algorithm that identified three symptoms unique to COVID-19 compared to the flu: ageusia — loss of the tongue’s taste function — shortness of breath, and anosmia, or loss of smell. The algorithm was developed in collaboration with two graduate students, Md Imrul Kaish and Md Jakir Hossain, at the University of Texas Rio Grande Valley.
“Much of the work using Google Trends for flu has focused on forecasting the flu season,” Papalexakis said. “We, on the other hand, used it to see if we could find a needle in a haystack: symptoms unique to COVID-19 among all the flu-like symptoms people search for.”
The researchers located symptoms on Google Trends for 2019 and 2020 and used a technique they called nonnegative discriminative analysis, or DNA, to extract terms that were unique to one dataset relative to the other.
“We assumed that symptom searches in 2019 would lead to influenza or other respiratory ailments, while searches for the same symptoms in 2020 could be either,” Chen said. “Using DNA, we were able to find the difference between the two datasets. This happened to be terms clinicians have already identified as unique to COVID-19, showing that our approach works.”
Papalexakis and Chen expect their work will help epidemiologists and other public health experts track and monitor COVID-19 using Google Trends as a proxy for hospital data.
“Google trends data is very noisy, but hospital data is not publicly available. People might search for symptoms because they are experiencing them or because they have heard of them and want to know more,” Papalexakis said. “Searches reflect interest in symptoms better than people actively experiencing them, but given the lack of other data, we think this tool could help researchers understand symptoms better.”
Chen said that the algorithm is simple and easy to implement as part of a potential tool that can help scientists researching other diseases learn about potential symptoms.
The paper, “COVID-19 or Flu? Discriminative Knowledge Discovery of COVID-19 Symptoms from Google Trends Data,” was presented at epiDAMIK 2021, a workshop on data mining for advancing epidemiological knowledge. The workshop was organized as part of the largest annual data science conference, the Association for Computing Machinery’s, or ACM, Special Interest Group on Knowledge Discovery and Data Mining. The paper is available here.
Papalexakis and UC Riverside doctoral student William Shiao are also developing a tool that not only identifies COVID-19 misinformation but shows why the information is flagged as false in relation to a database of scientific articles about research on coronaviruses.
Papalexakis and Shiao used 90,000 articles from the COVID-19 Open Research Dataset Challenge (CORD-19) prepared by the White House and a coalition of research groups, and collected 20,000 articles “in the wild” with misinformation about the novel coronavirus. Using a similarity matrix-based embedding method they called KI2TE, the articles were linked to a set of reference documents and interpreted. The documents used for reference were a set of academic papers on coronavirus research included in the CORD-19 dataset.
When tested on articles that had been labeled by humans as false or identified by Google Fact Check as false, their method not only correctly identified the false stories but also pointed to the scientific sources that corroborated the system’s decision.
“We are not interested in censoring what people see. We want to go beyond hiding something altogether or simply showing a warning label,” Papalexakis said. “We want to also show them sources to educate them.”
Although the tool developed by Papalexakis and Shiao is a prototype under active research development, it could eventually be incorporated into a smartphone app or into social media platforms like Facebook.
The results of this research were presented at the “Knowledge Graphs for Online Discourse Analysis” workshop organized as part of the ACM Web Conference, and the paper, “KI2TE: Knowledge-Infused InterpreTable Embeddings for COVID-19 Misinformation Detection,” is available here.